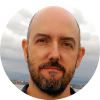
Javier Pascau
Javier Pascau is Full Professor in the Department of Bioengineering and Aerospace Engineering at Universidad Carlos III de Madrid. He received his degree on Telecommunication Engineering from Universidad Politécnica de Madrid in 1999, a Master in Biomedical Technology and Instrumentation in 2005 and his PhD from Universidad Politécnica de Madrid in 2006. He is part of the Biomedical Imaging and Instrumentation Group (BIIG, lab BIIG-IGT https://biig-igt.uc3m.es) at Universidad Carlos III de Madrid, and is also senior researcher at Instituto de Investigación Sanitaria Gregorio Marañón. Dr. Pascau has published works on medical image analysis with deep learning techniques, neuroimaging quantification, multimodal fusion, radiotherapy, molecular and functional imaging, high-resolution devices for animals, or information systems in radiology. He collaborates with the clinical departments of Traumatology, Surgery, Radiodiagnosis, Radiation Oncology or Gynecology at Hospital Gregorio Marañón or Clínica Universidad de Navarra, in both research and advisory tasks (selection of imaging equipment and RIS/PACS systems). His group implemented a surgical theater with specific hardware for image guided procedures inside the radiation therapy department at Hospital G. Marañón in Madrid to research in surgical navigation, intraoperative imaging with ultrasound or surface scanning, 3D printing or image registration, to personalize medical treatments. Dr Pascau lectures on Medical Image Processing in both undergraduate (Biomedical Engineering) and graduate levels (Masters in Health information Eng. and Clinical Eng.). He has been supervisor of 5 defended PhD theses and 4 ongoing. From 2016 to 2020 he was deputy director of the Biomedical Eng. Degree at Univ. Carlos III de Madrid.
Hands-on session: Image Guided Surgery
Image-Guided surgery (IGS) refers to clinical treatments where the physician uses tracked instruments, registered to preoperative or intraoperative images, to guide the procedure. IGS uses tracking systems of different types to obtain the position and orientation of specific surgical instruments. These tools are tracked in real-time with respect to the patient’s imaging studies during the intervention improving speed, security, efficacy, and surgical outcome. Tracking devices are an essential part of IGS. Optical tracking systems (OTS) or electromagnetic tracking systems (EMTS) are the most common, thanks to their versatility, size, and precision. OTS provide high tracking accuracy to any tool attached to spherical reflective markers, but need direct line-of-sight from the cameras to the instrument. On the other hand, EMTS overcome this limitation and are a good alternative when occlusions are inevitable or tracked tools are inside the patient. We can find optical tracking devices, such as Polaris Vega (Northern Digital Inc., ON, Canada), in many surgical navigation solutions such as StealthStation (Medtronic) or Curve Navigation (BrainLAB AG). These navigation systems are widely used in surgical treatments where rigid structures, such as bones, are involved because they ensure a low patient-to-image registration error, being neurosurgery the first procedure that benefited from this technology. Different open-source software proposals have allowed the easy introduction of IGS in the research context in the past years. PLUS toolkit, combined with OpenIGTLink, are good examples since they were implemented to manage interventional tool pose and image data from a wide range of tracking and imaging devices. This information can be live-streamed to software platforms such as 3D Slicer, where we also have access to state-of-the-art registration, segmentation, and visualization of medical images. In the first part of this practical session you will learn the basic algorithms and technologies involved in IGS, with examples of research and clinical applications. Then, during the tutorial, you will connect with an OTS to perform all the necessary steps to guide a brain tumor biopsy on a phantom. All the software tools required for the tutorial are open source, so you can take advantage of the learned features in your research applications.