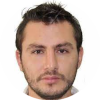
Adan Guerrero
Imaging has proven to be pivotal to tackle societal challenges. Cell biology discovery is closely intertwined with imaging and advances in microscopy technologies. Understanding the molecular architecture of nature requires advanced microscopy techniques which demand specialized infrastructure and knowledge. My scientific endeavor is rooted in unraveling nature at the nano/micro scales by means of using and developing imaging technologies. At the National Laboratory for Advanced Microscopy of Mexico at UNAM, we contribute to the development of optical nanoscopy by pioneering the development, implementation, and extensive use of super-resolution microscopy. I am convinced that international cooperation is an excellent mechanism to foster sustainability in advancing bioimaging in life sciences. Providing equal access to affordable training, eliminating gender and wealth disparities, and achieving universal access to a quality higher education stems societal development. I have an active role in paving the way for the development of the Mexican and Latin American bioimaging communities, with a major emphasis on establishing educational programs in bioimaging, aiming to create social interventions which foster development of humankind through empowering scientific discovery.
Optical Nanoscopy & Networking Initiatives on Bioimaging in Latin America
This lecture explores the recent advancements in optical nanoscopy techniques and their applications in bioimaging, with a particular emphasis on Latin America. The first section of the lecture delves into two major breakthroughs in optical nanoscopy: Fluorescence Fluctuation-based Super-resolution Microscopy (FF-SRM) and Mean-Shift Super Resolution (MSSR). FF-SRM encompasses a variety of image analysis techniques that use the statistical processing of fluorescence signal temporal variations to enhance spatial resolution. MSSR, a novel SRM algorithm based on the Mean Shift theory, surpasses the diffraction limit of light to extend the spatial resolution of single fluorescence images. The lecture will elucidate the fundamental concepts and principles behind these methods, their operational parameters, and offer guidance for their selection.
The second part of the lecture highlights educational and networking initiatives designed to promote the growth of bioimaging in Latin America. The Latin American Bioimaging platform aims to bolster the regional bioimaging community by offering training, resources, development opportunities, and partnerships. The lecture will examine three specific bioimaging initiatives in Mexico, including a decentralized educational workshop program, a visiting scientific program for imaging and biomedical scientists in Mexico and Argentina, and the development of a napari plugin for superresolution microscopy. These initiatives seek to foster collaboration, share best practices, enhance facility access, and broaden global access to bioimaging resources and expertise in the region.