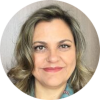
Letícia Rittner
Dr. Leticia Rittner is an Associate Professor (with tenure) at the School of Electrical and Computer Engineering, University of Campinas (Unicamp), Brazil. She is the co-founder and currently Director of the Medical Image Computing Laboratory (http://miclab.fee.unicamp.br/), and also part of the Brazilian Institute of Neuroscience and Neurotechnology (BRAINN). Dr. Rittner holds a bachelor’s degree in Electrical Engineering (1994), a Master in Business and Administration (1998), and a MSc (2004) and PhD (2009) in Computer Engineering from the University of Campinas. She held fellowships at the Montreal Neurological Institute, McGill University (Canada), and at the School of Medicine, University of Pennsylvania (USA). She was also a visiting Professor at Università degli Studi di Verona, Italy. Her research field is Medical Image Processing and Analysis, developing techniques based on machine learning, such as segmentation and characterization of anatomical structures, to support large-scale studies. Since 2012, she runs programming workshops for children and adolescents and has an active role in promoting gender diversity in the STEM areas. She is a member of the Brazilian Society of Biomedical Engineering (SBEB), of the International Photonic Society (SPIE) and a senior member of IEEE.
Deep Learning for medical image segmentation
Over the past few years, more and more researchers have joined the medical imaging processing and analysis field. Although most of the concepts related to image processing and deep learning have a vast bibliography and thousands of supporting materials, such as blogs, tutorials and commented code, it is not easy to find a summary of best practices related to a specific application, such as medical image segmentation.
This lecture will be divided in two parts: a theoretical introduction to the field of medical image segmentation and a hands-on session working on a real segmentation problem.
In the first part, I will share basic concepts for medical image segmentation that we have learned not only from books, but mostly from years of experience in the field. We will go through the basics of medical imaging, focusing on Magnetic Resonance (MR) and Computerized Tomography (CT), and the importance of good segmentation algorithms. Then, we will give an overview of deep learning algorithms, tools, and frameworks used in the specific field of medical segmentation tasks. Additionally, we will point out the main challenges and pitfalls one encounters while working in the field and will suggest best practices to overcome these challenges.
In the hands-on session, we will guide you while you try to solve a real-world segmentation problem, preparing the data and training your own deep learning model. By the end of the session, you will be able to evaluate your model and compare it to the state of the art methods.